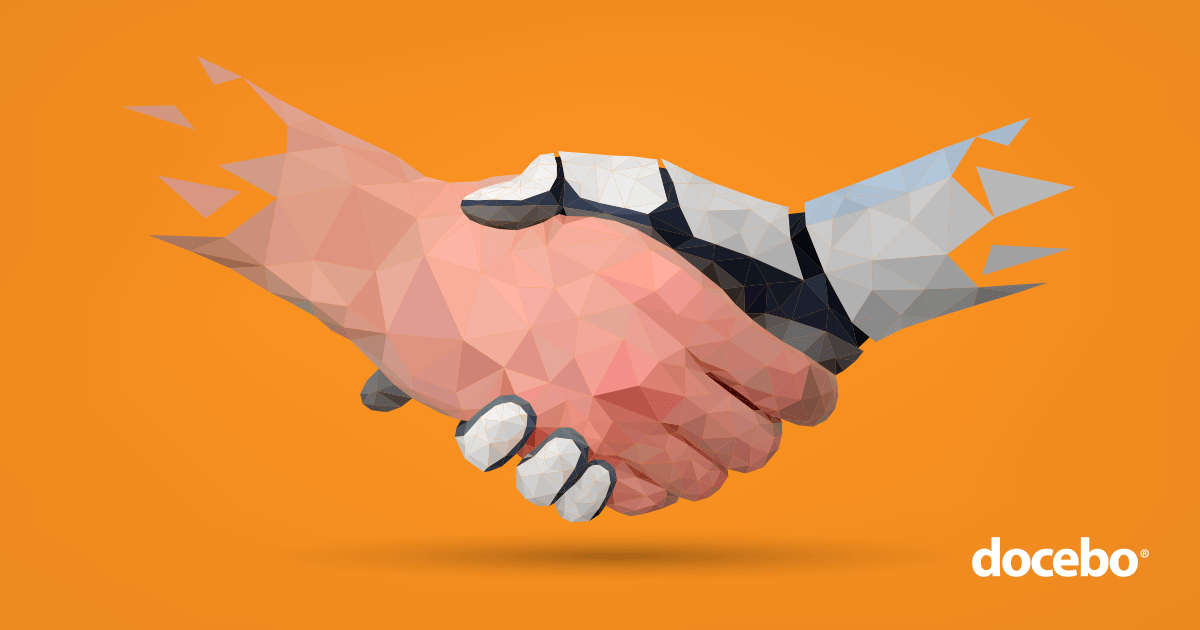
True learning personalization requires a deeper understanding of a learner’s role, depending on a deep understanding of behavioral patterns and real-time data to create highly contextual learning experiences, powered by the right technologies, that are more relevant, more engaging, and therefore, more effective.
In fact, only 10% of learning organizations are currently applying personalized learning strategically in their L&D activities, according to Brandon Hall Group, while, 77% of L&D professionals say personalization is vital to employee engagement, and 94% of businesses say personalization is key to their success.
Meanwhile, user engagement with content has gone down by 60% thanks to this thing called ‘information overload,’ causing more and more people to tune out messages that aren’t deeply relevant or positively impacting their lives.
Artificial Intelligence (AI) is a powerful tool that elevates human decision-making because there is no statistical model or algorithm that can substitute the intuition of a human being.
If we consider how AI works alongside you, it can:
- Analyze learner behavior to understand skill gaps and then present targeted recommendations.
- Automate content scheduling and delivery processes for admins.
- And ultimately, boost training ROI because less training time + greater personalization = better profit margins.
Tear a Page From Marketing’s Personalization Playbook
In our personal lives, personalization is overtaking nearly every facet, from how we watch movies and TV shows, to listening to music or podcasts, to the way we shop. And marketers are taking notice (and taking action): 75% of consumers will be more likely to purchase from someone whose offerings are personalized according to individual preferences.
For context, to understand the potential of these new features, let’s look at the marketing industry as an example.
Personalization is the activity of leveraging technology to incorporate personal and transactional information, such as name, title, organization, purchase history, etc. into external communications.
Yet, AI is capable of producing hyper-personalization, which uses behavioral and real-time data to create highly contextual communications that are more relevant. For example, let’s say you’re browsing for a new backpack on a retailer’s app for around 15 mins and then leave without purchasing.
Previous behavioral data reveals you’ve searched and purchased different types of bags before, with your activity spiking on Wednesday nights on a mobile device.
A hyper-personalized campaign would be to send a push notification to your mobile device on a Wednesday advertising a flash sale on bags.
Most companies operate in the standard “personalization” part of the personalization maturity curve; maybe a first name in there, or some segmentation based on broad demographics.
But companies like Amazon and Spotify have moved to hyper-personalization and beyond with predictive personalization, where AI & machine learning analyze a whole host of factors to provide recommendations.
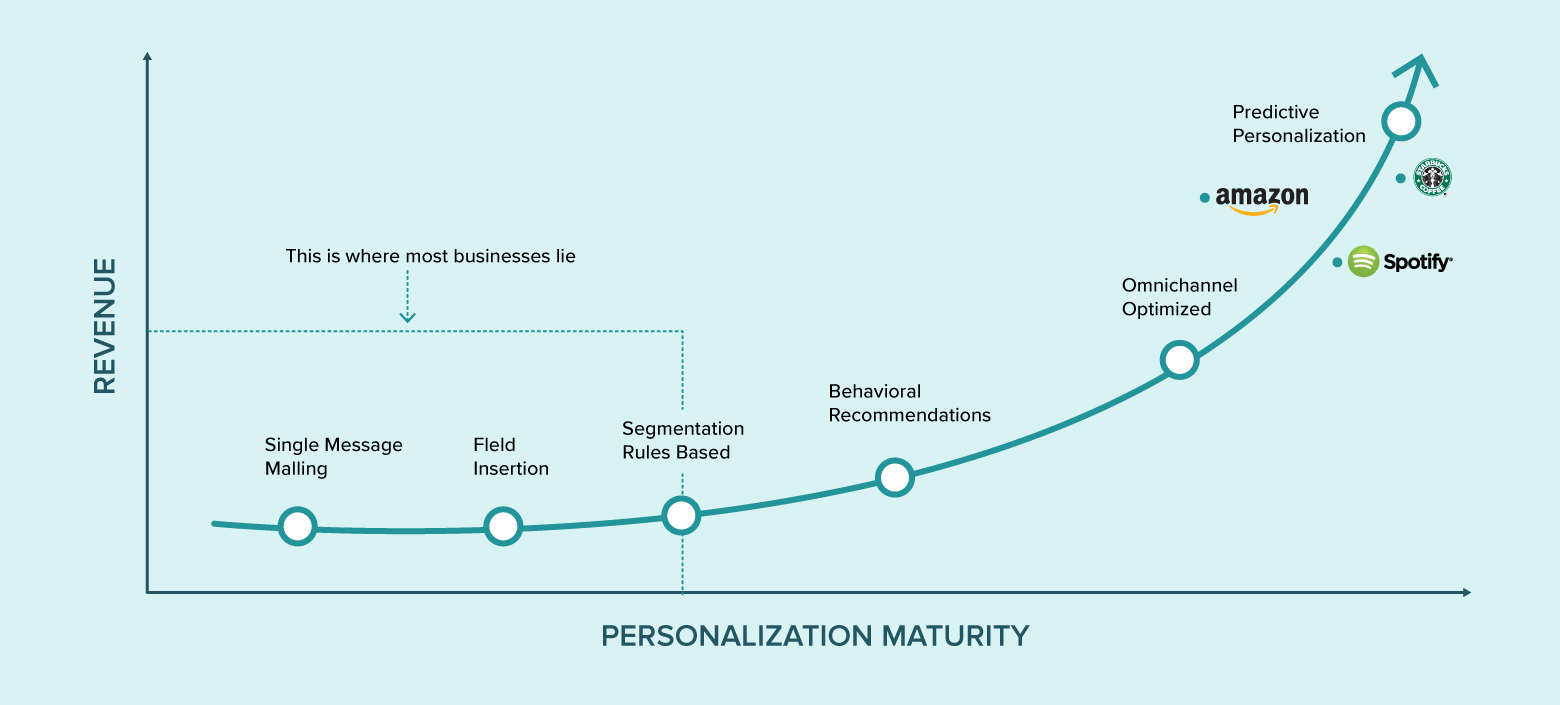
Applying Personalization to Learning
If we are to look at the learning industry, AI helps us understand learner behavior beyond traditional metrics, such as course completion While the number of learners who completed a course is very important, if taken as a metric alone, it doesn’t tell the full story. There might be some people that were interrupted and left the course in the middle of it and then came back later.
Or maybe some people took longer to complete it than others.
Artificial Intelligence not only understands when the course is completed but also each interaction a learner has while completing a course within their Learning Management System (LMS) or learning platform.
For example, are they asking a question or rating that particular course? What are they rating it? Did they pause for a while, and then continue?
All of these actions are tracked to supply the database required by machine learning models to understand difficult and complex correlations between courses and learners.
From that data, it extracts a better understanding of learner behavior.
While the go-to example for explaining the benefit of AI is content suggestions, the data used to produce those suggestions only tells half the story.
In learning, the AI requires a deeper data set to produce more relevant and effective results.
That being said, for the sake of providing a real-life example, let’s examine how Amazon is using AI and machine learning to power its recommendation engine – an algorithm called ‘item-to-item collaborative filtering’ that is generating 35% of the e-commerce giant’s conversions.
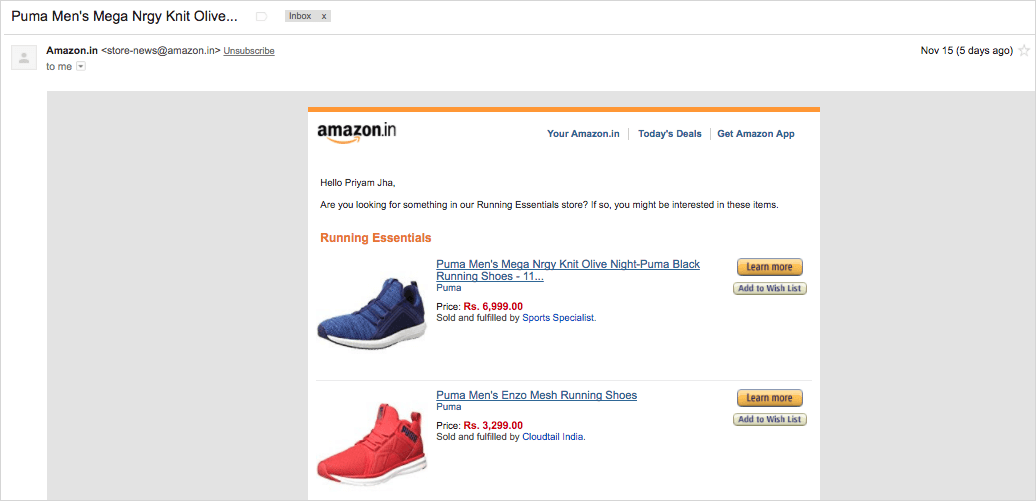
The Amazon Recommendation Engine
To generate personalized recommendations in its marketing emails, Amazon’s algorithm considers the following categories, based on a user’s interactions within their marketplace:
- Previously viewed items
- Product Saved to personal playlists
- Liked content
- Content consumed by similar learners
This tells a much deeper story about the kinds of recommendations the algorithms produces because they are based on more than just historical consumption data.
For context, Netflix and Spotify recommendations would only be generated by the “previously viewed items” category – or “you watched this/listened to this, so you might like this.”
So, in a learning context, to produce content suggestions or course enrolment, the algorithm could consider:
- previously viewed content (plus, which format that content is presented in)
- learning objects saved to personal channels/playlists
- “liked” content, either formal or learner-generated (social learning)
- content “liked”/consumed by others across the organizations w/ similar learner profiles
By understanding this, you’re able to make more informed decisions on how effective a certain type of course, with certain characteristics, will be for a specific learner.
You might uncover that a video is more suited to one learner, while slide presentations are more effective for another.
If we’re to consider the way organizations are investing in learning technology, this chart shows us that there is a shift occurring towards those that provide contextual and continuous learning support, while moving away from a focus on training delivery.

For example, “video” as a focus for training delivery is becoming saturated – this is because video is just that, a training delivery method.
And while video has proven itself to be useful as a tool that drives engagement, to be most effective, it must be facilitated in a way that each video-based learning asset is supported by continuous and contextual support mechanisms. For example, Artificial Intelligence and content curation that assist in developing the learning paths necessary to make learning more effective and aligned to business objectives.
Your learning platform should provide this kind of support.
Artificial Intelligence is one of the key tools that will drive major advances in the contextual support of enterprise learning because of its ability to determine and establish learning pathways that are adaptive and personalized to the unique needs of the learner.
Why Should You Care About Personalized Learning?
The psychology of personalization is rooted in an innate desire for control and power of choice. In a learning context, personalization produces the following outcomes (to name a couple):
Relevant Content = More Engagement: Data collection + AI serves up relevant recommendations.
Without engagement, you need to work extra hard to retain top performers. The key is showing and empowering the learner to see content that relates to where they are in their careers, and then deliver it in a way that enables them to retain it (boost interest, plus get them excited about applying the learning to their daily work)
It empowers learners to learn at a pace that suits them
Empowering personalized learning reflects that each employee has a unique role within your organization, producing higher-skilled and happier employees.
AI provides insights based on an enormous amount of data collected and analyzed by an LMS or learning platform, empowering the platform to develop a deeper understanding of learner behaviors and predict needs.
The result is content recommendations that are based on historical behaviors to produce more effective learning outcomes in the future, while fine-tuning via a feedback loop that provides continuous feedback on decisions the algorithm makes. AI understands if historical decisions were correct or not (then tweaks its own algorithm and provides accurate results).
In the context of personalized learning, micro-segmentation is key to creating learning that connects on a personalized level, providing a clearer picture of what each learner needs, what they value and how they behave.
AI would break data down to a point granular enough to understand what each learner needs most when they need it most, producing personalized learning experiences that are not only more effective but move the needle towards meeting organizational performance goals.
Support Personalized Learning Paths for Every Learner
Docebo’s 7.7 release introduces three new suggestion features powered by Artificial Intelligence, available to all users (learners, admins, managers), including:
Enrollment Suggestions for Admins. Superadmins will see a pre-populated list of users when enrolling users to a course, created by the platform’s AI, reducing the time needed to search for users who will benefit most from a specific course.
Formal Content Suggestions for Managers. Managers can see suggestions for courses in which they should enroll their team members from the My Team area of the platform, allowing them to take the learning progress of their teams into their own hands without waiting on Admin intervention.
Next Content Suggestions for Learners. For those using Coach & Share in Docebo, learners can take advantage of content suggestions that their peers are contributing in the platform, so they see the latest content to stay relevant and up-to-speed.
Support personalized learning for every learner – across your entire learning platform.